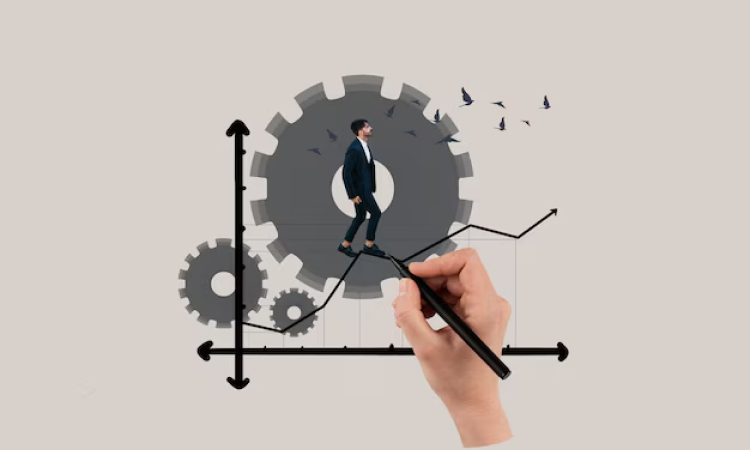
In the age of Industry 4.0, businesses are under immense pressure to optimize their operations, reduce downtime, and improve productivity. Manufacturing plants, power stations, transportation systems, and other industries that rely heavily on machinery and equipment face constant challenges in ensuring that their assets run smoothly. Unplanned downtime, costly repairs, and inefficiencies are the adversaries of profitability and sustainability.
Enter predictive maintenance—a powerful solution that leverages data, analytics, and machine learning to forecast equipment failures before they occur. By predicting when and where maintenance is needed, organizations can take proactive steps to minimize disruptions, extend the lifespan of assets, and enhance operational efficiency. In this blog, we’ll explore how predictive maintenance is revolutionizing industries, the technologies behind it, and the benefits it brings to businesses.
What is Predictive Maintenance?
Predictive maintenance (PdM) refers to the practice of using advanced data analytics, sensor technologies, and machine learning algorithms to predict when equipment will require maintenance. Unlike traditional maintenance strategies such as reactive maintenance (fixing equipment after a failure) or preventive maintenance (performing maintenance at set intervals regardless of condition), predictive maintenance is more dynamic and data-driven.
By analyzing real-time data from machinery, predictive maintenance systems can identify early signs of wear and tear or malfunctions, such as abnormal vibrations, temperature changes, or pressure fluctuations. This allows maintenance teams to address potential issues before they result in unplanned downtime, improving both asset reliability and operational efficiency.
How Predictive Maintenance Works
The core of predictive maintenance lies in data collection and analysis. Here's how it typically works:
Technologies Behind Predictive Maintenance
The effectiveness of predictive maintenance relies on several key technologies that work together to monitor, analyze, and predict equipment behavior. Here are some of the most important technologies driving PdM:
1. Internet of Things (IoT)
IoT devices are essential in predictive maintenance as they provide real-time data from machines and sensors. These devices can continuously monitor the health of equipment and transmit data to the cloud or local systems for analysis. The IoT enables predictive maintenance systems to access large amounts of real-time information, allowing for more accurate predictions.
2. Machine Learning and AI
Machine learning (ML) algorithms are at the heart of predictive maintenance. These algorithms learn from historical data and improve over time by identifying patterns or anomalies that humans may not immediately recognize. AI-powered systems can automatically adjust predictive models to account for new data, improving their ability to predict equipment failure with greater accuracy.
3. Data Analytics and Big Data
With the massive volume of data generated by IoT sensors and other sources, advanced data analytics tools help process and extract actionable insights. By leveraging big data, businesses can correlate data across various assets and components, creating more accurate predictive models that enhance maintenance planning.
4. Cloud Computing
Cloud computing offers the infrastructure to store and process vast amounts of data generated by predictive maintenance systems. Cloud platforms enable organizations to access real-time data, perform complex analytics, and collaborate across teams, all while reducing the need for costly on-site infrastructure.
5. Digital Twins
A digital twin is a virtual replica of physical assets, systems, or processes. Using sensor data and simulations, digital twins allow businesses to model the behavior of equipment in a virtual environment, helping to predict how it will perform over time. By monitoring the digital twin, maintenance teams can simulate potential failures and optimize maintenance schedules.
Benefits of Predictive Maintenance
The implementation of predictive maintenance offers numerous advantages to businesses across various industries. Here are some of the key benefits:
1. Reduced Downtime
Unplanned downtime is one of the most costly consequences of equipment failure. Predictive maintenance helps reduce this by enabling maintenance teams to perform repairs before a failure occurs. By catching issues early and scheduling maintenance proactively, businesses can significantly minimize unexpected downtime, leading to higher productivity.
2. Cost Savings
By predicting when maintenance is needed, businesses can avoid the high costs associated with emergency repairs and equipment replacement. Predictive maintenance allows for better resource allocation, as parts are replaced only when necessary, preventing unnecessary maintenance and extending the lifespan of assets.
Additionally, the reduction in downtime helps companies maintain steady production levels, preventing the loss of revenue from equipment breakdowns.
3. Improved Asset Lifespan
Routine preventive maintenance can be costly and often leads to replacing parts that haven’t yet worn out. With predictive maintenance, only parts showing signs of wear are replaced, extending the life of equipment and reducing capital expenditures on premature replacements.
4. Enhanced Safety
Predictive maintenance improves safety by addressing potential mechanical failures before they can cause accidents or dangerous conditions. By preventing equipment malfunctions, organizations can ensure a safer working environment for employees, reducing the risk of workplace injuries and accidents.
5. Optimized Resource Utilization
Rather than having maintenance teams check equipment on a fixed schedule, predictive maintenance allows for the scheduling of repairs and inspections only when necessary. This optimizes resource utilization by focusing efforts on the most critical tasks and preventing wasted time and labor.
6. Better Decision Making
With continuous access to real-time data and predictive insights, maintenance managers can make more informed decisions. This data-driven approach enables businesses to not only predict failures but also optimize operations across the organization, improving overall performance and efficiency.
Industries Benefiting from Predictive Maintenance
While predictive maintenance can benefit any industry relying on machinery, certain sectors are seeing particularly high levels of adoption:
1. Manufacturing
In manufacturing, equipment failure can lead to costly production delays and reduced output. Predictive maintenance helps manufacturers reduce downtime, optimize equipment usage, and improve overall production efficiency.
2. Energy
Power plants and energy providers rely heavily on critical equipment such as turbines and generators. Predictive maintenance helps identify potential failures in equipment before they cause disruptions in energy production, ensuring reliable power supply and preventing expensive repairs.
3. Transportation and Logistics
Airlines, shipping companies, and freight operators use predictive maintenance to maintain fleets of vehicles, vessels, and aircraft. By predicting mechanical failures before they happen, these organizations can reduce downtime, avoid costly repairs, and ensure that transportation services remain uninterrupted.
4. Oil and Gas
In the oil and gas industry, maintaining equipment such as pumps, compressors, and pipelines is critical to operations. Predictive maintenance allows companies to predict when key components are at risk of failure, ensuring smooth and continuous operation while minimizing safety risks.
5. Healthcare
Hospitals and healthcare providers rely on medical equipment such as MRI machines, ventilators, and diagnostic tools. Predictive maintenance ensures that these devices are always in optimal working condition, improving patient care and reducing the risk of equipment failure during critical procedures.
The Future of Predictive Maintenance
As technology continues to evolve, predictive maintenance will become even more advanced, with deeper integration of AI, machine learning, and IoT. Future developments in predictive maintenance may include:
Conclusion:
Unlocking Operational Efficiency with Predictive Maintenance
Predictive maintenance is transforming how industries manage their assets, moving from a reactive approach to a proactive one. By leveraging data and advanced analytics, organizations can predict failures, optimize maintenance schedules, and improve the overall reliability of their equipment.
The benefits of predictive maintenance—reduced downtime, cost savings, enhanced safety, and better resource utilization—are compelling for businesses looking to improve operational efficiency and remain competitive in a fast-paced market. As technology advances, predictive maintenance will continue to play a pivotal role in helping organizations operate smarter, faster, and more profitably.
Custom AI Models: Tailoring Solutions for Industry-Specific Needs
Read MoreRevolutionizing ERP: The Role of AI in Process Automation
Read Morewe’re committed to providing cutting-edge AI solutions that empower businesses to thrive in a fast-evolving digital landscape. Subscribe to our newsletter and stay informed on the latest advancements, industry insights, and how we can help transform your business with AI.