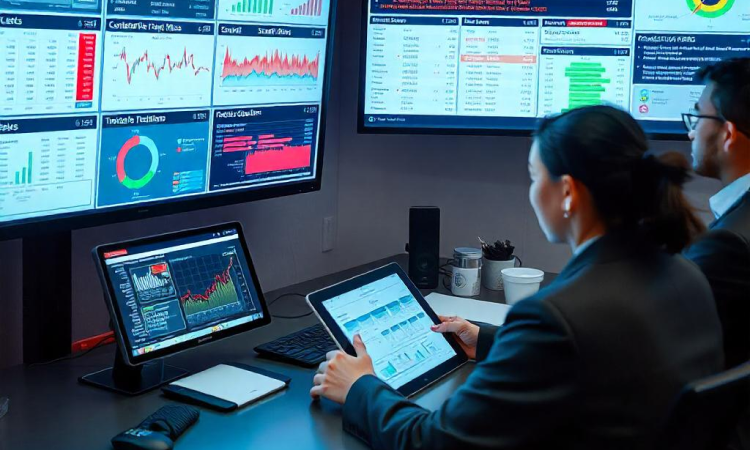
Fraud in banking has evolved alongside advancements in technology, becoming more sophisticated and harder to detect. Financial institutions face significant challenges in protecting customer assets and sensitive data from increasingly advanced fraudulent activities. In response, real-time fraud detection and prevention systems have become critical in the fight against fraud, enabling banks to identify and prevent fraudulent transactions as they happen.
By leveraging artificial intelligence (AI), machine learning (ML), and big data analytics, banks are transforming their approach to fraud prevention. These technologies provide the tools needed to detect suspicious activity quickly, reduce false positives, and ensure customers’ financial security.
In this article, we’ll dive into the importance of real-time fraud detection in banking, the role of AI and ML, and strategies that financial institutions can implement to stay ahead of fraud.
Fraud in the banking sector takes many forms, including:
As digital banking services, mobile transactions, and e-commerce grow, the opportunities for fraudsters to exploit vulnerabilities have multiplied. Fraud detection has evolved from relying on static, rule-based methods to more dynamic, data-driven systems capable of identifying irregular patterns and flagging suspicious activities in real time.
Real-time fraud detection systems continuously monitor and analyze customer transactions as they occur. By using AI and machine learning, these systems can:
Implementing a real-time fraud detection system offers several key benefits for banks:
The most obvious benefit of real-time fraud detection is the ability to stop fraudulent transactions before they are completed. For example, if a transaction deviates from a customer’s typical pattern, the system can temporarily freeze the account or alert the customer to confirm the transaction, effectively preventing further losses.
Traditional fraud detection systems often generate a high number of false positives, flagging legitimate transactions as fraudulent. This can result in frustrated customers, as well as operational inefficiencies. By using AI and machine learning, real-time systems are much more accurate at identifying true fraud risks while minimizing false alerts.
Real-time monitoring allows banks to act immediately, limiting the damage caused by fraud. Fraudsters often operate quickly, so a rapid response is essential in minimizing financial losses.
When customers feel their financial institution is actively protecting their assets, their trust and satisfaction increase. Real-time fraud detection systems that respond swiftly and accurately provide peace of mind to customers and enhance their overall experience.
Preventing fraud in real time can lead to significant cost savings. Financial losses from fraud are substantial, and implementing a proactive fraud detection system can reduce the impact of these losses.
AI and machine learning are the driving forces behind the evolution of real-time fraud detection systems. Here’s how they contribute to improved fraud prevention:
Machine learning algorithms analyze historical data to predict which transactions are likely to be fraudulent. By identifying subtle patterns and correlations, ML models can detect potential fraud before it occurs.
Unlike rule-based fraud detection, which requires predefined conditions, machine learning models continuously adapt to new threats. As fraudsters change tactics, ML algorithms learn and improve their predictive capabilities.
Fraud detection is no longer limited to credit card transactions. AI and machine learning can analyze transactions across multiple channels (online banking, mobile apps, ATMs, etc.) to detect fraud in any form.
Banks looking to implement or optimize real-time fraud detection systems should consider the following strategies:
While newer fraud detection solutions are highly advanced, many banks still rely on legacy systems. It’s important to integrate AI and machine learning models with existing infrastructure for seamless operation and improved fraud protection.
Banks can partner with specialized security providers to enhance their fraud detection capabilities. These providers offer ready-made AI and ML algorithms, as well as access to global fraud networks, which can help detect cross-border fraud.
Despite advanced fraud detection systems, customers must also play a role in preventing fraud. Banks should regularly educate customers on the importance of strong passwords, two-factor authentication, and vigilance against phishing scams.
While AI and ML are powerful tools for fraud detection, they should be part of a broader security strategy. Multi-layered security measures, including biometric authentication, encryption, and real-time transaction alerts, can help further reduce the risk of fraud.
Real-time fraud detection and prevention are no longer optional for banks—they are essential to maintaining customer trust and safeguarding financial assets. By leveraging AI, machine learning, and big data analytics, financial institutions can stay one step ahead of fraudsters, blocking suspicious transactions as they occur, and providing customers with a safer banking experience.
With fraud continuously evolving, banks must adopt dynamic and adaptive fraud prevention technologies to stay ahead of threats. Embracing real-time monitoring and cutting-edge security measures will not only reduce financial losses but also enhance customer loyalty and trust in the institution's ability to protect their assets.
we’re committed to providing cutting-edge AI solutions that empower businesses to thrive in a fast-evolving digital landscape. Subscribe to our newsletter and stay informed on the latest advancements, industry insights, and how we can help transform your business with AI.