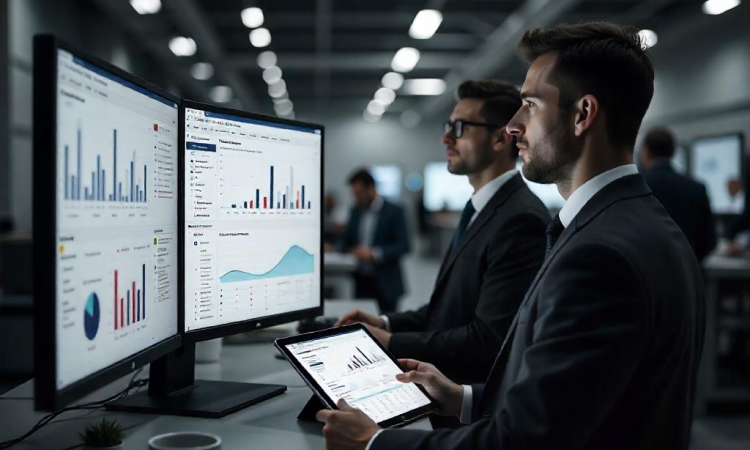
In the manufacturing industry, downtime is one of the most costly and disruptive issues. Every moment of production delay affects profitability, supply chain timelines, and overall operational efficiency. As manufacturing environments become increasingly complex with the integration of advanced machinery, equipment maintenance is critical for maintaining smooth operations. Traditionally, maintenance has been reactive or scheduled, leading to unnecessary repairs, unexpected breakdowns, and excessive maintenance costs. However, with the advent of predictive maintenance (PdM), manufacturers now have a powerful tool to minimize downtime, reduce costs, and improve productivity.
Predictive maintenance is an advanced strategy that leverages real-time data, machine learning algorithms, and IoT sensors to predict when equipment is likely to fail. Unlike traditional reactive maintenance, which only occurs after a failure happens, or preventive maintenance, which occurs at fixed intervals regardless of the machine's condition, predictive maintenance enables manufacturers to address issues before they result in failures.
This proactive approach utilizes historical data, equipment performance metrics, and environmental conditions to predict potential breakdowns and optimize maintenance schedules. By preventing unplanned downtimes and extending the life of equipment, predictive maintenance drives higher productivity and cost savings.
Predictive maintenance systems continuously monitor machines and equipment through sensors and data analytics. These sensors collect data on vibrations, temperature, pressure, humidity, and other factors affecting equipment performance. Advanced algorithms analyze this data in real time to detect abnormalities that may indicate an impending failure.
Early detection allows manufacturers to plan maintenance before the equipment fails, thus avoiding unscheduled downtime and emergency repairs. For example, if a bearing in a motor shows signs of wear, predictive maintenance can forecast its failure, enabling maintenance teams to replace it before it causes a more serious problem.
Rather than following rigid schedules, predictive maintenance schedules repairs based on the actual condition of the equipment. Data-driven insights allow manufacturers to perform maintenance tasks only when necessary, reducing the number of unnecessary interventions.
Maintenance teams are empowered to carry out repairs during planned downtimes or when production is least impacted, rather than during peak production hours. This reduces the likelihood of production interruptions and ensures that downtime is minimal.
Predictive maintenance can predict when a machine is nearing the end of its useful life or experiencing a malfunction that will soon lead to failure. By anticipating these issues, manufacturers can avoid the expensive and time-consuming process of emergency repairs or replacements.
Emergency repairs often come with a high price tag due to the need for expedited parts, overtime labor, and production delays. With predictive maintenance, issues can be addressed during planned maintenance windows, which significantly reduces the costs associated with emergency repairs.
Predictive maintenance enhances Overall Equipment Effectiveness (OEE), a key performance indicator used to measure the efficiency of manufacturing operations. OEE is calculated based on equipment availability, performance efficiency, and product quality. By reducing downtime through predictive insights, OEE can be maximized, ensuring that machines run at optimal levels for longer periods.
With fewer breakdowns and greater machine reliability, manufacturers experience higher production rates, better product quality, and reduced waste. This ultimately leads to higher throughput, improved profitability, and a stronger competitive position in the market.
Predictive maintenance systems provide valuable data on the operational health of machinery and equipment. Manufacturers can analyze this data to identify recurring problems, system weaknesses, or areas for process improvement. Machine learning algorithms continuously improve the accuracy of predictions by learning from past maintenance activities and failure patterns.
Data collected through predictive maintenance can drive improvements in equipment design, maintenance practices, and manufacturing processes. Over time, this leads to a more resilient production environment and a culture of continuous improvement.
IoT sensors are placed on critical machines to collect real-time data on factors such as vibration, temperature, pressure, and noise. These sensors continuously monitor equipment performance, providing the data needed for predictive analytics.
Predictive maintenance systems process vast amounts of data from sensors, equipment history, and external factors like environmental conditions. Big data analytics tools enable the system to identify trends, patterns, and anomalies in the data that signal the likelihood of failure.
Machine learning algorithms analyze historical data and learn from past failures to make more accurate predictions. These algorithms evolve over time, improving the system's ability to predict failures and optimize maintenance schedules.
Cloud-based platforms allow manufacturers to collect, store, and analyze data from anywhere, making it easier to manage predictive maintenance programs across multiple facilities and locations. Cloud systems offer scalability and flexibility, enabling businesses to handle large amounts of data and perform real-time analytics.
A large manufacturing plant that produces automotive parts was experiencing frequent downtime due to unexpected equipment failures, particularly in its assembly line robots. The plant implemented a predictive maintenance solution that used IoT sensors to monitor the robots' performance in real-time.
After a few months of data collection, the predictive maintenance system identified a recurring pattern of temperature spikes in the robot's motors, indicating that the motors were about to fail. The system alerted maintenance staff, who proactively scheduled a motor replacement during a planned downtime.
As a result, the plant reduced unplanned downtime by 30% and decreased emergency repair costs by 40%. In addition, the predictive maintenance system helped optimize the scheduling of maintenance tasks, leading to a 15% increase in overall equipment effectiveness (OEE).
Predictive maintenance is transforming the manufacturing industry by reducing downtime, improving operational efficiency, and cutting costs. By harnessing advanced technologies like IoT, machine learning, and big data analytics, manufacturers can move from reactive to proactive maintenance strategies, ultimately driving higher productivity and profitability.
As these technologies continue to evolve, predictive maintenance will become even more accurate and integrated into broader Industry 4.0 frameworks. Manufacturers that adopt predictive maintenance today will be better positioned to stay competitive in an increasingly dynamic and cost-conscious market, while also delivering improved product quality and customer satisfaction.
we’re committed to providing cutting-edge AI solutions that empower businesses to thrive in a fast-evolving digital landscape. Subscribe to our newsletter and stay informed on the latest advancements, industry insights, and how we can help transform your business with AI.